
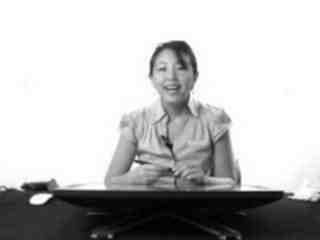
Dr. Ji Son
Central Tendency: Mean, Median, Mode
Slide Duration:Table of Contents
25m 31s
- Intro0:00
- Roadmap0:10
- Roadmap0:11
- Statistics0:35
- Statistics0:36
- Let's Think About High School Science1:12
- Measurement and Find Patterns (Mathematical Formula)1:13
- Statistics = Math of Distributions4:58
- Distributions4:59
- Problematic… but also GREAT5:58
- Statistics7:33
- How is It Different from Other Specializations in Mathematics?7:34
- Statistics is Fundamental in Natural and Social Sciences7:53
- Two Skills of Statistics8:20
- Description (Exploration)8:21
- Inference9:13
- Descriptive Statistics vs. Inferential Statistics: Apply to Distributions9:58
- Descriptive Statistics9:59
- Inferential Statistics11:05
- Populations vs. Samples12:19
- Populations vs. Samples: Is it the Truth?12:20
- Populations vs. Samples: Pros & Cons13:36
- Populations vs. Samples: Descriptive Values16:12
- Putting Together Descriptive/Inferential Stats & Populations/Samples17:10
- Putting Together Descriptive/Inferential Stats & Populations/Samples17:11
- Example 1: Descriptive Statistics vs. Inferential Statistics19:09
- Example 2: Descriptive Statistics vs. Inferential Statistics20:47
- Example 3: Sample, Parameter, Population, and Statistic21:40
- Example 4: Sample, Parameter, Population, and Statistic23:28
32m 14s
- Intro0:00
- Data0:09
- Data, Cases, Variables, and Values0:10
- Rows, Columns, and Cells2:03
- Example: Aircrafts3:52
- How Do We Get Data?5:38
- Research: Question and Hypothesis5:39
- Research Design7:11
- Measurement7:29
- Research Analysis8:33
- Research Conclusion9:30
- Types of Variables10:03
- Discrete Variables10:04
- Continuous Variables12:07
- Types of Measurements14:17
- Types of Measurements14:18
- Types of Measurements (Scales)17:22
- Nominal17:23
- Ordinal19:11
- Interval21:33
- Ratio24:24
- Example 1: Cases, Variables, Measurements25:20
- Example 2: Which Scale of Measurement is Used?26:55
- Example 3: What Kind of a Scale of Measurement is This?27:26
- Example 4: Discrete vs. Continuous Variables.30:31
8m 9s
- Intro0:00
- Before Visualizing Distribution0:10
- Excel0:11
- Excel: Organization0:45
- Workbook0:46
- Column x Rows1:50
- Tools: Menu Bar, Standard Toolbar, and Formula Bar3:00
- Excel + Data6:07
- Exce and Data6:08
39m 10s
- Intro0:00
- Roadmap0:08
- Data in Excel and Frequency Distributions0:09
- Raw Data to Frequency Tables0:42
- Raw Data to Frequency Tables0:43
- Frequency Tables: Using Formulas and Pivot Tables1:28
- Example 1: Number of Births7:17
- Example 2: Age Distribution20:41
- Example 3: Height Distribution27:45
- Example 4: Height Distribution of Males32:19
25m 29s
- Intro0:00
- Roadmap0:10
- Data in Excel, Frequency Distributions, and Features of Frequency Distributions0:11
- Example #11:35
- Uniform1:36
- Example #22:58
- Unimodal, Skewed Right, and Asymmetric2:59
- Example #36:29
- Bimodal6:30
- Example #4a8:29
- Symmetric, Unimodal, and Normal8:30
- Point of Inflection and Standard Deviation11:13
- Example #4b12:43
- Normal Distribution12:44
- Summary13:56
- Uniform, Skewed, Bimodal, and Normal13:57
- Sketch Problem 1: Driver's License17:34
- Sketch Problem 2: Life Expectancy20:01
- Sketch Problem 3: Telephone Numbers22:01
- Sketch Problem 4: Length of Time Used to Complete a Final Exam23:43
42m 42s
- Intro0:00
- Roadmap0:06
- Roadmap0:07
- Previously1:02
- Data, Frequency Table, and visualization1:03
- Dotplots1:22
- Dotplots Excel Example1:23
- Dotplots: Pros and Cons7:22
- Pros and Cons of Dotplots7:23
- Dotplots Excel Example Cont.9:07
- Histograms12:47
- Histograms Overview12:48
- Example of Histograms15:29
- Histograms: Pros and Cons31:39
- Pros31:40
- Cons32:31
- Frequency vs. Relative Frequency32:53
- Frequency32:54
- Relative Frequency33:36
- Example 1: Dotplots vs. Histograms34:36
- Example 2: Age of Pennies Dotplot36:21
- Example 3: Histogram of Mammal Speeds38:27
- Example 4: Histogram of Life Expectancy40:30
12m 23s
- Intro0:00
- Roadmap0:05
- Roadmap0:06
- What Sets Stemplots Apart?0:46
- Data Sets, Dotplots, Histograms, and Stemplots0:47
- Example 1: What Do Stemplots Look Like?1:58
- Example 2: Back-to-Back Stemplots5:00
- Example 3: Quiz Grade Stemplot7:46
- Example 4: Quiz Grade & Afterschool Tutoring Stemplot9:56
22m 49s
- Intro0:00
- Roadmap0:05
- Roadmap0:08
- Review of Frequency Distributions0:44
- Y-axis and X-axis0:45
- Types of Frequency Visualizations Covered so Far2:16
- Introduction to Bar Graphs4:07
- Example 1: Bar Graph5:32
- Example 1: Bar Graph5:33
- Do Shapes, Center, and Spread of Distributions Apply to Bar Graphs?11:07
- Do Shapes, Center, and Spread of Distributions Apply to Bar Graphs?11:08
- Example 2: Create a Frequency Visualization for Gender14:02
- Example 3: Cases, Variables, and Frequency Visualization16:34
- Example 4: What Kind of Graphs are Shown Below?19:29
38m 50s
- Intro0:00
- Roadmap0:07
- Roadmap0:08
- Central Tendency 10:56
- Way to Summarize a Distribution of Scores0:57
- Mode1:32
- Median2:02
- Mean2:36
- Central Tendency 23:47
- Mode3:48
- Median4:20
- Mean5:25
- Summation Symbol6:11
- Summation Symbol6:12
- Population vs. Sample10:46
- Population vs. Sample10:47
- Excel Examples15:08
- Finding Mode, Median, and Mean in Excel15:09
- Median vs. Mean21:45
- Effect of Outliers21:46
- Relationship Between Parameter and Statistic22:44
- Type of Measurements24:00
- Which Distributions to Use With24:55
- Example 1: Mean25:30
- Example 2: Using Summation Symbol29:50
- Example 3: Average Calorie Count32:50
- Example 4: Creating an Example Set35:46
42m 40s
- Intro0:00
- Roadmap0:05
- Roadmap0:06
- Variability (or Spread)0:45
- Variability (or Spread)0:46
- Things to Think About5:45
- Things to Think About5:46
- Range, Quartiles and Interquartile Range6:37
- Range6:38
- Interquartile Range8:42
- Interquartile Range Example10:58
- Interquartile Range Example10:59
- Variance and Standard Deviation12:27
- Deviations12:28
- Sum of Squares14:35
- Variance16:55
- Standard Deviation17:44
- Sum of Squares (SS)18:34
- Sum of Squares (SS)18:35
- Population vs. Sample SD22:00
- Population vs. Sample SD22:01
- Population vs. Sample23:20
- Mean23:21
- SD23:51
- Example 1: Find the Mean and Standard Deviation of the Variable Friends in the Excel File27:21
- Example 2: Find the Mean and Standard Deviation of the Tagged Photos in the Excel File35:25
- Example 3: Sum of Squares38:58
- Example 4: Standard Deviation41:48
57m 15s
- Intro0:00
- Roadmap0:06
- Roadmap0:07
- Summarizing Distributions0:37
- Shape, Center, and Spread0:38
- 5 Number Summary1:14
- Boxplot: Visualizing 5 Number Summary3:37
- Boxplot: Visualizing 5 Number Summary3:38
- Boxplots on Excel9:01
- Using 'Stocks' and Using Stacked Columns9:02
- Boxplots on Excel Example10:14
- When are Boxplots Useful?32:14
- Pros32:15
- Cons32:59
- How to Determine Outlier Status33:24
- Rule of Thumb: Upper Limit33:25
- Rule of Thumb: Lower Limit34:16
- Signal Outliers in an Excel Data File Using Conditional Formatting34:52
- Modified Boxplot48:38
- Modified Boxplot48:39
- Example 1: Percentage Values & Lower and Upper Whisker49:10
- Example 2: Boxplot50:10
- Example 3: Estimating IQR From Boxplot53:46
- Example 4: Boxplot and Missing Whisker54:35
41m 51s
- Intro0:00
- Roadmap0:16
- Roadmap0:17
- Skewness Concept1:09
- Skewness Concept1:10
- Calculating Skewness3:26
- Calculating Skewness3:27
- Interpreting Skewness7:36
- Interpreting Skewness7:37
- Excel Example8:49
- Kurtosis Concept20:29
- Kurtosis Concept20:30
- Calculating Kurtosis24:17
- Calculating Kurtosis24:18
- Interpreting Kurtosis29:01
- Leptokurtic29:35
- Mesokurtic30:10
- Platykurtic31:06
- Excel Example32:04
- Example 1: Shape of Distribution38:28
- Example 2: Shape of Distribution39:29
- Example 3: Shape of Distribution40:14
- Example 4: Kurtosis41:10
34m 33s
- Intro0:00
- Roadmap0:13
- Roadmap0:14
- What is a Normal Distribution0:44
- The Normal Distribution As a Theoretical Model0:45
- Possible Range of Probabilities3:05
- Possible Range of Probabilities3:06
- What is a Normal Distribution5:07
- Can Be Described By5:08
- Properties5:49
- 'Same' Shape: Illusion of Different Shape!7:35
- 'Same' Shape: Illusion of Different Shape!7:36
- Types of Problems13:45
- Example: Distribution of SAT Scores13:46
- Shape Analogy19:48
- Shape Analogy19:49
- Example 1: The Standard Normal Distribution and Z-Scores22:34
- Example 2: The Standard Normal Distribution and Z-Scores25:54
- Example 3: Sketching and Normal Distribution28:55
- Example 4: Sketching and Normal Distribution32:32
41m 44s
- Intro0:00
- Roadmap0:06
- Roadmap0:07
- A Family of Distributions0:28
- Infinite Set of Distributions0:29
- Transforming Normal Distributions to 'Standard' Normal Distribution1:04
- Normal Distribution vs. Standard Normal Distribution2:58
- Normal Distribution vs. Standard Normal Distribution2:59
- Z-Score, Raw Score, Mean, & SD4:08
- Z-Score, Raw Score, Mean, & SD4:09
- Weird Z-Scores9:40
- Weird Z-Scores9:41
- Excel16:45
- For Normal Distributions16:46
- For Standard Normal Distributions19:11
- Excel Example20:24
- Types of Problems25:18
- Percentage Problem: P(x)25:19
- Raw Score and Z-Score Problems26:28
- Standard Deviation Problems27:01
- Shape Analogy27:44
- Shape Analogy27:45
- Example 1: Deaths Due to Heart Disease vs. Deaths Due to Cancer28:24
- Example 2: Heights of Male College Students33:15
- Example 3: Mean and Standard Deviation37:14
- Example 4: Finding Percentage of Values in a Standard Normal Distribution37:49
55m 44s
- Intro0:00
- Roadmap0:15
- Roadmap0:16
- Frequency vs. Cumulative Frequency0:56
- Frequency vs. Cumulative Frequency0:57
- Frequency vs. Cumulative Frequency4:32
- Frequency vs. Cumulative Frequency Cont.4:33
- Calculus in Brief6:21
- Derivative-Integral Continuum6:22
- PDF10:08
- PDF for Standard Normal Distribution10:09
- PDF for Normal Distribution14:32
- Integral of PDF = CDF21:27
- Integral of PDF = CDF21:28
- Example 1: Cumulative Frequency Graph23:31
- Example 2: Mean, Standard Deviation, and Probability24:43
- Example 3: Mean and Standard Deviation35:50
- Example 4: Age of Cars49:32
47m 19s
- Intro0:00
- Roadmap0:04
- Roadmap0:05
- Previous Visualizations0:30
- Frequency Distributions0:31
- Compare & Contrast2:26
- Frequency Distributions Vs. Scatterplots2:27
- Summary Values4:53
- Shape4:54
- Center & Trend6:41
- Spread & Strength8:22
- Univariate & Bivariate10:25
- Example Scatterplot10:48
- Shape, Trend, and Strength10:49
- Positive and Negative Association14:05
- Positive and Negative Association14:06
- Linearity, Strength, and Consistency18:30
- Linearity18:31
- Strength19:14
- Consistency20:40
- Summarizing a Scatterplot22:58
- Summarizing a Scatterplot22:59
- Example 1: Gapminder.org, Income x Life Expectancy26:32
- Example 2: Gapminder.org, Income x Infant Mortality36:12
- Example 3: Trend and Strength of Variables40:14
- Example 4: Trend, Strength and Shape for Scatterplots43:27
32m 2s
- Intro0:00
- Roadmap0:05
- Roadmap0:06
- Linear Equations0:34
- Linear Equations: y = mx + b0:35
- Rough Line5:16
- Rough Line5:17
- Regression - A 'Center' Line7:41
- Reasons for Summarizing with a Regression Line7:42
- Predictor and Response Variable10:04
- Goal of Regression12:29
- Goal of Regression12:30
- Prediction14:50
- Example: Servings of Mile Per Year Shown By Age14:51
- Intrapolation17:06
- Extrapolation17:58
- Error in Prediction20:34
- Prediction Error20:35
- Residual21:40
- Example 1: Residual23:34
- Example 2: Large and Negative Residual26:30
- Example 3: Positive Residual28:13
- Example 4: Interpret Regression Line & Extrapolate29:40
56m 36s
- Intro0:00
- Roadmap0:13
- Roadmap0:14
- Best Fit0:47
- Best Fit0:48
- Sum of Squared Errors (SSE)1:50
- Sum of Squared Errors (SSE)1:51
- Why Squared?3:38
- Why Squared?3:39
- Quantitative Properties of Regression Line4:51
- Quantitative Properties of Regression Line4:52
- So How do we Find Such a Line?6:49
- SSEs of Different Line Equations & Lowest SSE6:50
- Carl Gauss' Method8:01
- How Do We Find Slope (b1)11:00
- How Do We Find Slope (b1)11:01
- Hoe Do We Find Intercept15:11
- Hoe Do We Find Intercept15:12
- Example 1: Which of These Equations Fit the Above Data Best?17:18
- Example 2: Find the Regression Line for These Data Points and Interpret It26:31
- Example 3: Summarize the Scatterplot and Find the Regression Line.34:31
- Example 4: Examine the Mean of Residuals43:52
43m 58s
- Intro0:00
- Roadmap0:05
- Roadmap0:06
- Summarizing a Scatterplot Quantitatively0:47
- Shape0:48
- Trend1:11
- Strength: Correlation ®1:45
- Correlation Coefficient ( r )2:30
- Correlation Coefficient ( r )2:31
- Trees vs. Forest11:59
- Trees vs. Forest12:00
- Calculating r15:07
- Average Product of z-scores for x and y15:08
- Relationship between Correlation and Slope21:10
- Relationship between Correlation and Slope21:11
- Example 1: Find the Correlation between Grams of Fat and Cost24:11
- Example 2: Relationship between r and b130:24
- Example 3: Find the Regression Line33:35
- Example 4: Find the Correlation Coefficient for this Set of Data37:37
52m 52s
- Intro0:00
- Roadmap0:07
- Roadmap0:08
- R-squared0:44
- What is the Meaning of It? Why Squared?0:45
- Parsing Sum of Squared (Parsing Variability)2:25
- SST = SSR + SSE2:26
- What is SST and SSE?7:46
- What is SST and SSE?7:47
- r-squared18:33
- Coefficient of Determination18:34
- If the Correlation is Strong…20:25
- If the Correlation is Strong…20:26
- If the Correlation is Weak…22:36
- If the Correlation is Weak…22:37
- Example 1: Find r-squared for this Set of Data23:56
- Example 2: What Does it Mean that the Simple Linear Regression is a 'Model' of Variance?33:54
- Example 3: Why Does r-squared Only Range from 0 to 137:29
- Example 4: Find the r-squared for This Set of Data39:55
27m 8s
- Intro0:00
- Roadmap0:05
- Roadmap0:06
- Why Transform?0:26
- Why Transform?0:27
- Shape-preserving vs. Shape-changing Transformations5:14
- Shape-preserving = Linear Transformations5:15
- Shape-changing Transformations = Non-linear Transformations6:20
- Common Shape-Preserving Transformations7:08
- Common Shape-Preserving Transformations7:09
- Common Shape-Changing Transformations8:59
- Powers9:00
- Logarithms9:39
- Change Just One Variable? Both?10:38
- Log-log Transformations10:39
- Log Transformations14:38
- Example 1: Create, Graph, and Transform the Data Set15:19
- Example 2: Create, Graph, and Transform the Data Set20:08
- Example 3: What Kind of Model would You Choose for this Data?22:44
- Example 4: Transformation of Data25:46
54m 44s
- Intro0:00
- Roadmap0:05
- Roadmap0:06
- Descriptive vs. Inferential Statistics1:04
- Descriptive Statistics: Data Exploration1:05
- Example2:03
- To tackle Generalization…4:31
- Generalization4:32
- Sampling6:06
- 'Good' Sample6:40
- Defining Samples and Populations8:55
- Population8:56
- Sample11:16
- Why Use Sampling?13:09
- Why Use Sampling?13:10
- Goal of Sampling: Avoiding Bias15:04
- What is Bias?15:05
- Where does Bias Come from: Sampling Bias17:53
- Where does Bias Come from: Response Bias18:27
- Sampling Bias: Bias from Bas Sampling Methods19:34
- Size Bias19:35
- Voluntary Response Bias21:13
- Convenience Sample22:22
- Judgment Sample23:58
- Inadequate Sample Frame25:40
- Response Bias: Bias from 'Bad' Data Collection Methods28:00
- Nonresponse Bias29:31
- Questionnaire Bias31:10
- Incorrect Response or Measurement Bias37:32
- Example 1: What Kind of Biases?40:29
- Example 2: What Biases Might Arise?44:46
- Example 3: What Kind of Biases?48:34
- Example 4: What Kind of Biases?51:43
14m 25s
- Intro0:00
- Roadmap0:05
- Roadmap0:06
- Biased vs. Unbiased Sampling Methods0:32
- Biased Sampling0:33
- Unbiased Sampling1:13
- Probability Sampling Methods2:31
- Simple Random2:54
- Stratified Random Sampling4:06
- Cluster Sampling5:24
- Two-staged Sampling6:22
- Systematic Sampling7:25
- Example 1: Which Type(s) of Sampling was this?8:33
- Example 2: Describe How to Take a Two-Stage Sample from this Book10:16
- Example 3: Sampling Methods11:58
- Example 4: Cluster Sample Plan12:48
53m 54s
- Intro0:00
- Roadmap0:06
- Roadmap0:07
- Descriptive vs. Inferential Statistics0:51
- Descriptive Statistics: Data Exploration0:52
- Inferential Statistics1:02
- Variables and Relationships1:44
- Variables1:45
- Relationships2:49
- Not Every Type of Study is an Experiment…4:16
- Category I - Descriptive Study4:54
- Category II - Correlational Study5:50
- Category III - Experimental, Quasi-experimental, Non-experimental6:33
- Category III7:42
- Experimental, Quasi-experimental, and Non-experimental7:43
- Why CAN'T the Other Strategies Determine Causation?10:18
- Third-variable Problem10:19
- Directionality Problem15:49
- What Makes Experiments Special?17:54
- Manipulation17:55
- Control (and Comparison)21:58
- Methods of Control26:38
- Holding Constant26:39
- Matching29:11
- Random Assignment31:48
- Experiment Terminology34:09
- 'true' Experiment vs. Study34:10
- Independent Variable (IV)35:16
- Dependent Variable (DV)35:45
- Factors36:07
- Treatment Conditions36:23
- Levels37:43
- Confounds or Extraneous Variables38:04
- Blind38:38
- Blind Experiments38:39
- Double-blind Experiments39:29
- How Categories Relate to Statistics41:35
- Category I - Descriptive Study41:36
- Category II - Correlational Study42:05
- Category III - Experimental, Quasi-experimental, Non-experimental42:43
- Example 1: Research Design43:50
- Example 2: Research Design47:37
- Example 3: Research Design50:12
- Example 4: Research Design52:00
41m 31s
- Intro0:00
- Roadmap0:06
- Roadmap0:07
- Experimental Designs0:51
- Experimental Designs: Manipulation & Control0:52
- Two Types of Variability2:09
- Between Treatment Variability2:10
- Within Treatment Variability3:31
- Updated Goal of Experimental Design5:47
- Updated Goal of Experimental Design5:48
- Example: Drugs and Driving6:56
- Example: Drugs and Driving6:57
- Different Types of Random Assignment11:27
- All Experiments11:28
- Completely Random Design12:02
- Randomized Block Design13:19
- Randomized Block Design15:48
- Matched Pairs Design15:49
- Repeated Measures Design19:47
- Between-subject Variable vs. Within-subject Variable22:43
- Completely Randomized Design22:44
- Repeated Measures Design25:03
- Example 1: Design a Completely Random, Matched Pair, and Repeated Measures Experiment26:16
- Example 2: Block Design31:41
- Example 3: Completely Randomized Designs35:11
- Example 4: Completely Random, Matched Pairs, or Repeated Measures Experiments?39:01
37m 52s
- Intro0:00
- Roadmap0:07
- Roadmap0:08
- Why is Probability Involved in Statistics0:48
- Probability0:49
- Can People Tell the Difference between Cheap and Gourmet Coffee?2:08
- Taste Test with Coffee Drinkers3:37
- If No One can Actually Taste the Difference3:38
- If Everyone can Actually Taste the Difference5:36
- Creating a Probability Model7:09
- Creating a Probability Model7:10
- D'Alembert vs. Necker9:41
- D'Alembert vs. Necker9:42
- Problem with D'Alembert's Model13:29
- Problem with D'Alembert's Model13:30
- Covering Entire Sample Space15:08
- Fundamental Principle of Counting15:09
- Where Do Probabilities Come From?22:54
- Observed Data, Symmetry, and Subjective Estimates22:55
- Checking whether Model Matches Real World24:27
- Law of Large Numbers24:28
- Example 1: Law of Large Numbers27:46
- Example 2: Possible Outcomes30:43
- Example 3: Brands of Coffee and Taste33:25
- Example 4: How Many Different Treatments are there?35:33
20m 29s
- Intro0:00
- Roadmap0:08
- Roadmap0:09
- Disjoint Events0:41
- Disjoint Events0:42
- Meaning of 'or'2:39
- In Regular Life2:40
- In Math/Statistics/Computer Science3:10
- Addition Rule for Disjoin Events3:55
- If A and B are Disjoint: P (A and B)3:56
- If A and B are Disjoint: P (A or B)5:15
- General Addition Rule5:41
- General Addition Rule5:42
- Generalized Addition Rule8:31
- If A and B are not Disjoint: P (A or B)8:32
- Example 1: Which of These are Mutually Exclusive?10:50
- Example 2: What is the Probability that You will Have a Combination of One Heads and Two Tails?12:57
- Example 3: Engagement Party15:17
- Example 4: Home Owner's Insurance18:30
57m 19s
- Intro0:00
- Roadmap0:05
- Roadmap0:06
- 'or' vs. 'and' vs. Conditional Probability1:07
- 'or' vs. 'and' vs. Conditional Probability1:08
- 'and' vs. Conditional Probability5:57
- P (M or L)5:58
- P (M and L)8:41
- P (M|L)11:04
- P (L|M)12:24
- Tree Diagram15:02
- Tree Diagram15:03
- Defining Conditional Probability22:42
- Defining Conditional Probability22:43
- Common Contexts for Conditional Probability30:56
- Medical Testing: Positive Predictive Value30:57
- Medical Testing: Sensitivity33:03
- Statistical Tests34:27
- Example 1: Drug and Disease36:41
- Example 2: Marbles and Conditional Probability40:04
- Example 3: Cards and Conditional Probability45:59
- Example 4: Votes and Conditional Probability50:21
24m 27s
- Intro0:00
- Roadmap0:05
- Roadmap0:06
- Independent Events & Conditional Probability0:26
- Non-independent Events0:27
- Independent Events2:00
- Non-independent and Independent Events3:08
- Non-independent and Independent Events3:09
- Defining Independent Events5:52
- Defining Independent Events5:53
- Multiplication Rule7:29
- Previously…7:30
- But with Independent Evens8:53
- Example 1: Which of These Pairs of Events are Independent?11:12
- Example 2: Health Insurance and Probability15:12
- Example 3: Independent Events17:42
- Example 4: Independent Events20:03
56m 45s
- Intro0:00
- Roadmap0:08
- Roadmap0:09
- Sampling vs. Probability0:57
- Sampling0:58
- Missing1:30
- What is Missing?3:06
- Insight: Probability Distributions5:26
- Insight: Probability Distributions5:27
- What is a Probability Distribution?7:29
- From Sample Spaces to Probability Distributions8:44
- Sample Space8:45
- Probability Distribution of the Sum of Two Die11:16
- The Random Variable17:43
- The Random Variable17:44
- Expected Value21:52
- Expected Value21:53
- Example 1: Probability Distributions28:45
- Example 2: Probability Distributions35:30
- Example 3: Probability Distributions43:37
- Example 4: Probability Distributions47:20
53m 41s
- Intro0:00
- Roadmap0:06
- Roadmap0:07
- Discrete vs. Continuous Random Variables1:04
- Discrete vs. Continuous Random Variables1:05
- Mean and Variance Review4:44
- Mean: Sample, Population, and Probability Distribution4:45
- Variance: Sample, Population, and Probability Distribution9:12
- Example Situation14:10
- Example Situation14:11
- Some Special Cases…16:13
- Some Special Cases…16:14
- Linear Transformations19:22
- Linear Transformations19:23
- What Happens to Mean and Variance of the Probability Distribution?20:12
- n Independent Values of X25:38
- n Independent Values of X25:39
- Compare These Two Situations30:56
- Compare These Two Situations30:57
- Two Random Variables, X and Y32:02
- Two Random Variables, X and Y32:03
- Example 1: Expected Value & Variance of Probability Distributions35:35
- Example 2: Expected Values & Standard Deviation44:17
- Example 3: Expected Winnings and Standard Deviation48:18
55m 15s
- Intro0:00
- Roadmap0:05
- Roadmap0:06
- Discrete Probability Distributions1:42
- Discrete Probability Distributions1:43
- Binomial Distribution2:36
- Binomial Distribution2:37
- Multiplicative Rule Review6:54
- Multiplicative Rule Review6:55
- How Many Outcomes with k 'Successes'10:23
- Adults and Bachelor's Degree: Manual List of Outcomes10:24
- P (X=k)19:37
- Putting Together # of Outcomes with the Multiplicative Rule19:38
- Expected Value and Standard Deviation in a Binomial Distribution25:22
- Expected Value and Standard Deviation in a Binomial Distribution25:23
- Example 1: Coin Toss33:42
- Example 2: College Graduates38:03
- Example 3: Types of Blood and Probability45:39
- Example 4: Expected Number and Standard Deviation51:11
48m 17s
- Intro0:00
- Roadmap0:08
- Roadmap0:09
- Probability Distributions vs. Sampling Distributions0:55
- Probability Distributions vs. Sampling Distributions0:56
- Same Logic3:55
- Logic of Probability Distribution3:56
- Example: Rolling Two Die6:56
- Simulating Samples9:53
- To Come Up with Probability Distributions9:54
- In Sampling Distributions11:12
- Connecting Sampling and Research Methods with Sampling Distributions12:11
- Connecting Sampling and Research Methods with Sampling Distributions12:12
- Simulating a Sampling Distribution14:14
- Experimental Design: Regular Sleep vs. Less Sleep14:15
- Logic of Sampling Distributions23:08
- Logic of Sampling Distributions23:09
- General Method of Simulating Sampling Distributions25:38
- General Method of Simulating Sampling Distributions25:39
- Questions that Remain28:45
- Questions that Remain28:46
- Example 1: Mean and Standard Error of Sampling Distribution30:57
- Example 2: What is the Best Way to Describe Sampling Distributions?37:12
- Example 3: Matching Sampling Distributions38:21
- Example 4: Mean and Standard Error of Sampling Distribution41:51
1h 8m 48s
- Intro0:00
- Roadmap0:05
- Roadmap0:06
- Special Case of General Method for Simulating a Sampling Distribution1:53
- Special Case of General Method for Simulating a Sampling Distribution1:54
- Computer Simulation3:43
- Using Simulations to See Principles behind Shape of SDoM15:50
- Using Simulations to See Principles behind Shape of SDoM15:51
- Conditions17:38
- Using Simulations to See Principles behind Center (Mean) of SDoM20:15
- Using Simulations to See Principles behind Center (Mean) of SDoM20:16
- Conditions: Does n Matter?21:31
- Conditions: Does Number of Simulation Matter?24:37
- Using Simulations to See Principles behind Standard Deviation of SDoM27:13
- Using Simulations to See Principles behind Standard Deviation of SDoM27:14
- Conditions: Does n Matter?34:45
- Conditions: Does Number of Simulation Matter?36:24
- Central Limit Theorem37:13
- SHAPE38:08
- CENTER39:34
- SPREAD39:52
- Comparing Population, Sample, and SDoM43:10
- Comparing Population, Sample, and SDoM43:11
- Answering the 'Questions that Remain'48:24
- What Happens When We Don't Know What the Population Looks Like?48:25
- Can We Have Sampling Distributions for Summary Statistics Other than the Mean?49:42
- How Do We Know whether a Sample is Sufficiently Unlikely?53:36
- Do We Always Have to Simulate a Large Number of Samples in Order to get a Sampling Distribution?54:40
- Example 1: Mean Batting Average55:25
- Example 2: Mean Sampling Distribution and Standard Error59:07
- Example 3: Sampling Distribution of the Mean1:01:04
54m 37s
- Intro0:00
- Roadmap0:06
- Roadmap0:07
- Intro to Sampling Distribution of Sample Proportions (SDoSP)0:51
- Categorical Data (Examples)0:52
- Wish to Estimate Proportion of Population from Sample…2:00
- Notation3:34
- Population Proportion and Sample Proportion Notations3:35
- What's the Difference?9:19
- SDoM vs. SDoSP: Type of Data9:20
- SDoM vs. SDoSP: Shape11:24
- SDoM vs. SDoSP: Center12:30
- SDoM vs. SDoSP: Spread15:34
- Binomial Distribution vs. Sampling Distribution of Sample Proportions19:14
- Binomial Distribution vs. SDoSP: Type of Data19:17
- Binomial Distribution vs. SDoSP: Shape21:07
- Binomial Distribution vs. SDoSP: Center21:43
- Binomial Distribution vs. SDoSP: Spread24:08
- Example 1: Sampling Distribution of Sample Proportions26:07
- Example 2: Sampling Distribution of Sample Proportions37:58
- Example 3: Sampling Distribution of Sample Proportions44:42
- Example 4: Sampling Distribution of Sample Proportions45:57
42m 53s
- Intro0:00
- Roadmap0:06
- Roadmap0:07
- Inferential Statistics0:50
- Inferential Statistics0:51
- Two Problems with This Picture…3:20
- Two Problems with This Picture…3:21
- Solution: Confidence Intervals (CI)4:59
- Solution: Hypotheiss Testing (HT)5:49
- Which Parameters are Known?6:45
- Which Parameters are Known?6:46
- Confidence Interval - Goal7:56
- When We Don't Know m but know s7:57
- When We Don't Know18:27
- When We Don't Know m nor s18:28
- Example 1: Confidence Intervals26:18
- Example 2: Confidence Intervals29:46
- Example 3: Confidence Intervals32:18
- Example 4: Confidence Intervals38:31
1h 2m 6s
- Intro0:00
- Roadmap0:04
- Roadmap0:05
- When to Use z vs. t?1:07
- When to Use z vs. t?1:08
- What is z and t?3:02
- z-score and t-score: Commonality3:03
- z-score and t-score: Formulas3:34
- z-score and t-score: Difference5:22
- Why not z? (Why t?)7:24
- Why not z? (Why t?)7:25
- But Don't Worry!15:13
- Gossett and t-distributions15:14
- Rules of t Distributions17:05
- t-distributions are More Normal as n Gets Bigger17:06
- t-distributions are a Family of Distributions18:55
- Degrees of Freedom (df)20:02
- Degrees of Freedom (df)20:03
- t Family of Distributions24:07
- t Family of Distributions : df = 2 , 4, and 6024:08
- df = 6029:16
- df = 229:59
- How to Find It?31:01
- 'Student's t-distribution' or 't-distribution'31:02
- Excel Example33:06
- Example 1: Which Distribution Do You Use? Z or t?45:26
- Example 2: Friends on Facebook47:41
- Example 3: t Distributions52:15
- Example 4: t Distributions , confidence interval, and mean55:59
1h 6m 33s
- Intro0:00
- Roadmap0:06
- Roadmap0:07
- Issues to Overcome in Inferential Statistics1:35
- Issues to Overcome in Inferential Statistics1:36
- What Happens When We Don't Know What the Population Looks Like?2:57
- How Do We Know whether a sample is Sufficiently Unlikely3:43
- Hypothesizing a Population6:44
- Hypothesizing a Population6:45
- Null Hypothesis8:07
- Alternative Hypothesis8:56
- Hypotheses11:58
- Hypotheses11:59
- Errors in Hypothesis Testing14:22
- Errors in Hypothesis Testing14:23
- Steps of Hypothesis Testing21:15
- Steps of Hypothesis Testing21:16
- Single Sample HT ( When Sigma Available)26:08
- Example: Average Facebook Friends26:09
- Step127:08
- Step 227:58
- Step 328:17
- Step 432:18
- Single Sample HT (When Sigma Not Available)36:33
- Example: Average Facebook Friends36:34
- Step1: Hypothesis Testing36:58
- Step 2: Significance Level37:25
- Step 3: Decision Stage37:40
- Step 4: Sample41:36
- Sigma and p-value45:04
- Sigma and p-value45:05
- On tailed vs. Two Tailed Hypotheses45:51
- Example 1: Hypothesis Testing48:37
- Example 2: Heights of Women in the US57:43
- Example 3: Select the Best Way to Complete This Sentence1:03:23
55m 14s
- Intro0:00
- Roadmap0:14
- Roadmap0:15
- One Mean vs. Two Means1:17
- One Mean vs. Two Means1:18
- Notation2:41
- A Sample! A Set!2:42
- Mean of X, Mean of Y, and Difference of Two Means3:56
- SE of X4:34
- SE of Y6:28
- Sampling Distribution of the Difference between Two Means (SDoD)7:48
- Sampling Distribution of the Difference between Two Means (SDoD)7:49
- Rules of the SDoD (similar to CLT!)15:00
- Mean for the SDoD Null Hypothesis15:01
- Standard Error17:39
- When can We Construct a CI for the Difference between Two Means?21:28
- Three Conditions21:29
- Finding CI23:56
- One Mean CI23:57
- Two Means CI25:45
- Finding t29:16
- Finding t29:17
- Interpreting CI30:25
- Interpreting CI30:26
- Better Estimate of s (s pool)34:15
- Better Estimate of s (s pool)34:16
- Example 1: Confidence Intervals42:32
- Example 2: SE of the Difference52:36
50m
- Intro0:00
- Roadmap0:06
- Roadmap0:07
- The Goal of Hypothesis Testing0:56
- One Sample and Two Samples0:57
- Sampling Distribution of the Difference between Two Means (SDoD)3:42
- Sampling Distribution of the Difference between Two Means (SDoD)3:43
- Rules of the SDoD (Similar to CLT!)6:46
- Shape6:47
- Mean for the Null Hypothesis7:26
- Standard Error for Independent Samples (When Variance is Homogenous)8:18
- Standard Error for Independent Samples (When Variance is not Homogenous)9:25
- Same Conditions for HT as for CI10:08
- Three Conditions10:09
- Steps of Hypothesis Testing11:04
- Steps of Hypothesis Testing11:05
- Formulas that Go with Steps of Hypothesis Testing13:21
- Step 113:25
- Step 214:18
- Step 315:00
- Step 416:57
- Example 1: Hypothesis Testing for the Difference of Two Independent Means18:47
- Example 2: Hypothesis Testing for the Difference of Two Independent Means33:55
- Example 3: Hypothesis Testing for the Difference of Two Independent Means44:22
1h 14m 11s
- Intro0:00
- Roadmap0:09
- Roadmap0:10
- The Goal of Hypothesis Testing1:27
- One Sample and Two Samples1:28
- Independent Samples vs. Paired Samples3:16
- Independent Samples vs. Paired Samples3:17
- Which is Which?5:20
- Independent SAMPLES vs. Independent VARIABLES7:43
- independent SAMPLES vs. Independent VARIABLES7:44
- T-tests Always…10:48
- T-tests Always…10:49
- Notation for Paired Samples12:59
- Notation for Paired Samples13:00
- Steps of Hypothesis Testing for Paired Samples16:13
- Steps of Hypothesis Testing for Paired Samples16:14
- Rules of the SDoD (Adding on Paired Samples)18:03
- Shape18:04
- Mean for the Null Hypothesis18:31
- Standard Error for Independent Samples (When Variance is Homogenous)19:25
- Standard Error for Paired Samples20:39
- Formulas that go with Steps of Hypothesis Testing22:59
- Formulas that go with Steps of Hypothesis Testing23:00
- Confidence Intervals for Paired Samples30:32
- Confidence Intervals for Paired Samples30:33
- Example 1: Confidence Intervals & Hypothesis Testing for the Difference of Two Paired Means32:28
- Example 2: Confidence Intervals & Hypothesis Testing for the Difference of Two Paired Means44:02
- Example 3: Confidence Intervals & Hypothesis Testing for the Difference of Two Paired Means52:23
31m 27s
- Intro0:00
- Roadmap0:18
- Roadmap0:19
- Errors and Relationship to HT and the Sample Statistic?1:11
- Errors and Relationship to HT and the Sample Statistic?1:12
- Instead of a Box…Distributions!7:00
- One Sample t-test: Friends on Facebook7:01
- Two Sample t-test: Friends on Facebook13:46
- Usually, Lots of Overlap between Null and Alternative Distributions16:59
- Overlap between Null and Alternative Distributions17:00
- How Distributions and 'Box' Fit Together22:45
- How Distributions and 'Box' Fit Together22:46
- Example 1: Types of Errors25:54
- Example 2: Types of Errors27:30
- Example 3: What is the Danger of the Type I Error?29:38
44m 41s
- Intro0:00
- Roadmap0:05
- Roadmap0:06
- Distance between Distributions: Sample t0:49
- Distance between Distributions: Sample t0:50
- Problem with Distance in Terms of Standard Error2:56
- Problem with Distance in Terms of Standard Error2:57
- Test Statistic (t) vs. Effect Size (d or g)4:38
- Test Statistic (t) vs. Effect Size (d or g)4:39
- Rules of Effect Size6:09
- Rules of Effect Size6:10
- Why Do We Need Effect Size?8:21
- Tells You the Practical Significance8:22
- HT can be Deceiving…10:25
- Important Note10:42
- What is Power?11:20
- What is Power?11:21
- Why Do We Need Power?14:19
- Conditional Probability and Power14:20
- Power is:16:27
- Can We Calculate Power?19:00
- Can We Calculate Power?19:01
- How Does Alpha Affect Power?20:36
- How Does Alpha Affect Power?20:37
- How Does Effect Size Affect Power?25:38
- How Does Effect Size Affect Power?25:39
- How Does Variability and Sample Size Affect Power?27:56
- How Does Variability and Sample Size Affect Power?27:57
- How Do We Increase Power?32:47
- Increasing Power32:48
- Example 1: Effect Size & Power35:40
- Example 2: Effect Size & Power37:38
- Example 3: Effect Size & Power40:55
24m 46s
- Intro0:00
- Roadmap0:04
- Roadmap0:05
- Z- & T-statistic and Their Distribution0:34
- Z- & T-statistic and Their Distribution0:35
- F-statistic4:55
- The F Ration ( the Variance Ratio)4:56
- F-distribution12:29
- F-distribution12:30
- s and p-value15:00
- s and p-value15:01
- Example 1: Why Does F-distribution Stop At 0 But Go On Until Infinity?18:33
- Example 2: F-distributions19:29
- Example 3: F-distributions and Heights21:29
1h 9m 25s
- Intro0:00
- Roadmap0:05
- Roadmap0:06
- The Limitations of t-tests1:12
- The Limitations of t-tests1:13
- Two Major Limitations of Many t-tests3:26
- Two Major Limitations of Many t-tests3:27
- Ronald Fisher's Solution… F-test! New Null Hypothesis4:43
- Ronald Fisher's Solution… F-test! New Null Hypothesis (Omnibus Test - One Test to Rule Them All!)4:44
- Analysis of Variance (ANoVA) Notation7:47
- Analysis of Variance (ANoVA) Notation7:48
- Partitioning (Analyzing) Variance9:58
- Total Variance9:59
- Within-group Variation14:00
- Between-group Variation16:22
- Time out: Review Variance & SS17:05
- Time out: Review Variance & SS17:06
- F-statistic19:22
- The F Ratio (the Variance Ratio)19:23
- S²bet = SSbet / dfbet22:13
- What is This?22:14
- How Many Means?23:20
- So What is the dfbet?23:38
- So What is SSbet?24:15
- S²w = SSw / dfw26:05
- What is This?26:06
- How Many Means?27:20
- So What is the dfw?27:36
- So What is SSw?28:18
- Chart of Independent Samples ANOVA29:25
- Chart of Independent Samples ANOVA29:26
- Example 1: Who Uploads More Photos: Unknown Ethnicity, Latino, Asian, Black, or White Facebook Users?35:52
- Hypotheses35:53
- Significance Level39:40
- Decision Stage40:05
- Calculate Samples' Statistic and p-Value44:10
- Reject or Fail to Reject H055:54
- Example 2: ANOVA with Independent Samples58:21
1h 15m 13s
- Intro0:00
- Roadmap0:05
- Roadmap0:06
- The Limitations of t-tests0:36
- Who Uploads more Pictures and Which Photo-Type is Most Frequently Used on Facebook?0:37
- ANOVA (F-test) to the Rescue!5:49
- Omnibus Hypothesis5:50
- Analyze Variance7:27
- Independent Samples vs. Repeated Measures9:12
- Same Start9:13
- Independent Samples ANOVA10:43
- Repeated Measures ANOVA12:00
- Independent Samples ANOVA16:00
- Same Start: All the Variance Around Grand Mean16:01
- Independent Samples16:23
- Repeated Measures ANOVA18:18
- Same Start: All the Variance Around Grand Mean18:19
- Repeated Measures18:33
- Repeated Measures F-statistic21:22
- The F Ratio (The Variance Ratio)21:23
- S²bet = SSbet / dfbet23:07
- What is This?23:08
- How Many Means?23:39
- So What is the dfbet?23:54
- So What is SSbet?24:32
- S² resid = SS resid / df resid25:46
- What is This?25:47
- So What is SS resid?26:44
- So What is the df resid?27:36
- SS subj and df subj28:11
- What is This?28:12
- How Many Subject Means?29:43
- So What is df subj?30:01
- So What is SS subj?30:09
- SS total and df total31:42
- What is This?31:43
- What is the Total Number of Data Points?32:02
- So What is df total?32:34
- so What is SS total?32:47
- Chart of Repeated Measures ANOVA33:19
- Chart of Repeated Measures ANOVA: F and Between-samples Variability33:20
- Chart of Repeated Measures ANOVA: Total Variability, Within-subject (case) Variability, Residual Variability35:50
- Example 1: Which is More Prevalent on Facebook: Tagged, Uploaded, Mobile, or Profile Photos?40:25
- Hypotheses40:26
- Significance Level41:46
- Decision Stage42:09
- Calculate Samples' Statistic and p-Value46:18
- Reject or Fail to Reject H057:55
- Example 2: Repeated Measures ANOVA58:57
- Example 3: What's the Problem with a Bunch of Tiny t-tests?1:13:59
58m 23s
- Intro0:00
- Roadmap0:05
- Roadmap0:06
- Where Does the Chi-Square Test Belong?0:50
- Where Does the Chi-Square Test Belong?0:51
- A New Twist on HT: Goodness-of-Fit7:23
- HT in General7:24
- Goodness-of-Fit HT8:26
- Hypotheses about Proportions12:17
- Null Hypothesis12:18
- Alternative Hypothesis13:23
- Example14:38
- Chi-Square Statistic17:52
- Chi-Square Statistic17:53
- Chi-Square Distributions24:31
- Chi-Square Distributions24:32
- Conditions for Chi-Square28:58
- Condition 128:59
- Condition 230:20
- Condition 330:32
- Condition 431:47
- Example 1: Chi-Square Goodness-of-Fit Test32:23
- Example 2: Chi-Square Goodness-of-Fit Test44:34
- Example 3: Which of These Statements Describe Properties of the Chi-Square Goodness-of-Fit Test?56:06
51m 36s
- Intro0:00
- Roadmap0:09
- Roadmap0:10
- Goodness-of-Fit vs. Homogeneity1:13
- Goodness-of-Fit HT1:14
- Homogeneity2:00
- Analogy2:38
- Hypotheses About Proportions5:00
- Null Hypothesis5:01
- Alternative Hypothesis6:11
- Example6:33
- Chi-Square Statistic10:12
- Same as Goodness-of-Fit Test10:13
- Set Up Data12:28
- Setting Up Data Example12:29
- Expected Frequency16:53
- Expected Frequency16:54
- Chi-Square Distributions & df19:26
- Chi-Square Distributions & df19:27
- Conditions for Test of Homogeneity20:54
- Condition 120:55
- Condition 221:39
- Condition 322:05
- Condition 422:23
- Example 1: Chi-Square Test of Homogeneity22:52
- Example 2: Chi-Square Test of Homogeneity32:10
18m 11s
- Intro0:00
- Roadmap0:07
- Roadmap0:08
- The Statistical Tests (HT) We've Covered0:28
- The Statistical Tests (HT) We've Covered0:29
- Organizing the Tests We've Covered…1:08
- One Sample: Continuous DV and Categorical DV1:09
- Two Samples: Continuous DV and Categorical DV5:41
- More Than Two Samples: Continuous DV and Categorical DV8:21
- The Following Data: OK Cupid10:10
- The Following Data: OK Cupid10:11
- Example 1: Weird-MySpace-Angle Profile Photo10:38
- Example 2: Geniuses12:30
- Example 3: Promiscuous iPhone Users13:37
- Example 4: Women, Aging, and Messaging16:07
For more information, please see full course syllabus of Statistics
Statistics Central Tendency: Mean, Median, Mode
In this lesson, we are going to be talking about central tendency, mean, median, and mode. Mean, median, and mode are what most people think about when they think about statistics, especially descriptive statistics. We are going to be talking about these three measures of central tendency. Then we are going to spend a little bit more time on mean. We are going to review the summation symbol Sigma (Σ) and we are also going to talk about the different formulas for population mean versus sample mean. Then we are going to talk about some Excel examples and finally we are going to compare these measures of central tendency.
Share this knowledge with your friends!
Copy & Paste this embed code into your website’s HTML
Please ensure that your website editor is in text mode when you paste the code.(In Wordpress, the mode button is on the top right corner.)
- - Allow users to view the embedded video in full-size.
0 answers
Post by Paulette Jones on May 8, 2013
Thanks for your help. You're a terrific teacher. :-)
0 answers
Post by DIntre Smith on August 21, 2012
Keep up the good work! Ever thought about teaching the Probability P/1 Actuary exam?
0 answers
Post by Johnnie Brown on March 26, 2012
You are a God send!!!!
0 answers
Post by Ryan Mulligan on January 26, 2012
Amazing teacher, Taking this course at University and our Russian prof is horrible. This series saved me for Midterms... Cheers!
0 answers
Post by munir eldeeb on February 8, 2011
your explanation is good, but your sloppy on things, like look at that S at 6:50